Responsible DS + AI Speaker Series: Maarten Sap, Allen Institute for AI
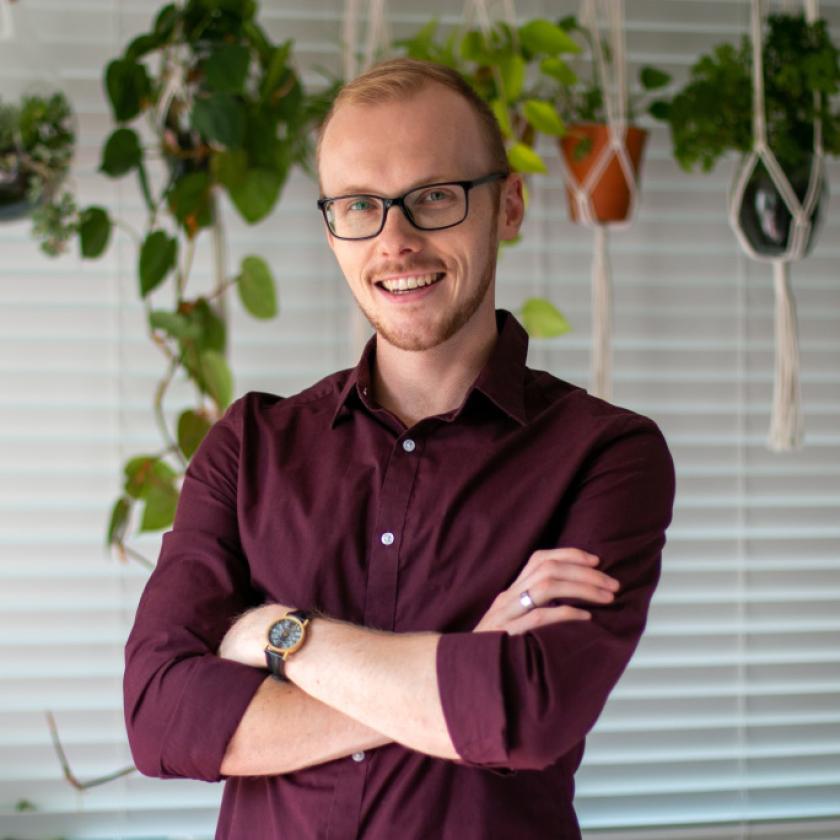
Maarten Sap, postdoc/young investigator at the Allen Institute for AI, will present "Detecting and Rewriting Socially Biased Language."
Maarten Sap is a postdoc/young investigator at the Allen Institute for AI (AI2) working on project Mosaic and will be starting as an assistant professor at CMU's LTI department. His research focuses on endowing NLP systems with social intelligence and social commonsense and understanding social inequality and bias in language. He received his PhD from the University of Washington, where he was advised by Noah Smith and Yejin Choi. He interned at AI2, working on social commonsense reasoning, and at Microsoft Research, working on deep learning models for understanding human cognition.
Abstract: Language has the power to reinforce stereotypes and project social biases onto others, either through overt hate or subtle biases. Accounting for this toxicity and social bias in language is crucial for natural language processing (NLP) systems to be safely and ethically deployed in the world. In this talk, I will first analyze a failure case of automatic hate speech detection, in which we find that models tend to flag speech by African Americans as toxic more often than by others. We trace the origins of the biases back to the annotated datasets, and show that we can reduce these biases, by making a tweet's dialect more explicit during the annotation process. Then, as an alternative to binary hate speech detection, I will present Social Bias Frames, a new structured formalism for distilling biased implications of language. Using a new corpus of 150k structured annotations, we show that models can learn to reason about high-level offensiveness of statements, but struggle to explain why a statement might be harmful. Finally, I will introduce PowerTransformer, a new unsupervised model for controllable debiasing of text through the lens of connotation frames of power and agency. With this model, we show that subtle gender biases in how characters are portrayed in stories and movies can be mitigated through automatic rewriting. I will conclude with future directions for better reasoning about toxicity and social biases in language.
Selected publications:
Sap, M., Card, D., Gabriel, S., Choi, Y., & Smith, N. A. (2019). The Risk of Racial Bias in Hate Speech Detection. In Proceedings of the 57th annual meeting of the association for computational linguistics (pp. 1668-1678).
Sap, M., Gabriel, S., Qin, L., Jurafsky, D., Smith, N. A., & Choi, Y. (2020). Social Bias Frames: Reasoning about Social and Power Implications of Language. In Proceedings of the 58th Annual Meeting of the Association for Computational Linguistics (pp. 5477-5490).
Ma, X., Sap, M., Rashkin, H., & Choi, Y. (2020). PowerTransformer: Unsupervised controllable revision for biased language correction. In Proceedings of the 2020 Conference on Empirical Methods in Natural Language Processing (EMNLP) (pp. 7426-7441).
Questions? Contact Janet Eke or Kanyao Han
The Responsible Data Science and AI Speaker Series discusses topics such as equity, fairness, biases, ethics, and privacy. The presentations and discussions take place on Fridays, 9-10 am Central Time, on Zoom. This series is organized by Associate Professor Jana Diesner and supported by the Center for Informatics Research in Science and Scholarship (CIRSS) and the School of Information Sciences at the University of Illinois Urbana-Champaign.
If you are interested in this speaker series, please subscribe to our speaker series calendar: Google Calendar or Outlook Calendar.
This event is sponsored by Center for Informatics Research in Science and Scholarship