CFG Seminar: Nigel Bosch
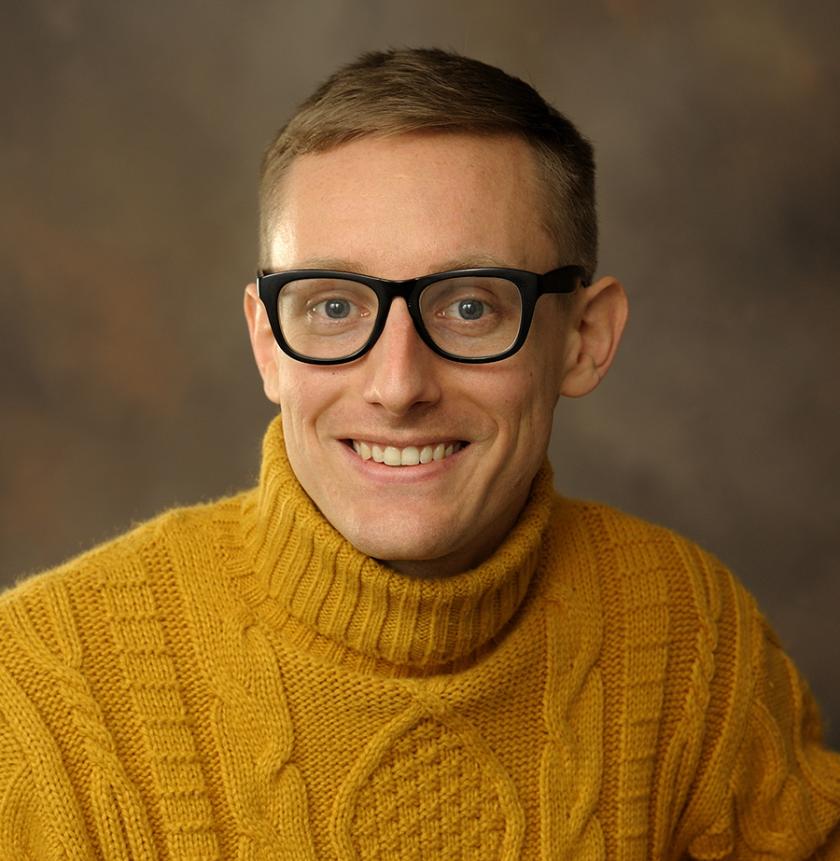
All are welcome to attend the Conceptual Foundation Group's (CFG) seminar, where Assistant Professor Nigel Bosch will present his recent work on the topic of "Utilizing Machine Learning to Model Conditional Average Treatment Effects (CATEs)."
Abstract:
In research and in everyday life we often seek to make causal inferences via various types of natural experiments and randomized controlled trials. Causal inferences typically concern the causal effect on some outcome across all "units," such as people, institutions, or whatever is supposed to be influenced. However, that mean effect (i.e., the "average treatment effect"), while important, may mask interesting variability. Some units may be more affected than others in a systematic fashion; for example, perhaps a scholarship program has an especially positive impact on graduation rates for one group of students but less so for their peers. In this talk I will describe some methods that use machine learning to model the heterogeneity in causal effects, or "conditional average treatment effects" (CATEs). Machine learning can capture conditional effects even in the presence of nonlinearity and interactions between subgroups. Finally, I will describe my ongoing work to discover CATEs in situations where they may not be known in advance, which requires interpretable machine learning methods.
Reading suggestions:
Athey, S., & Imbens, G. (2016). Recursive partitioning for heterogeneous causal effects. Proceedings of the National Academy of Sciences, 113(27), 7353-7360. https://www.pnas.org/doi/full/10.1073/pnas.1510489113
Davis, J. M., & Heller, S. B. (2020). Rethinking the benefits of youth employment programs: The heterogeneous effects of summer jobs. Review of economics and statistics, 102(4), 664-677. https://direct.mit.edu/rest/article-abstract/102/4/664/96778/Rethinking-the-Benefits-of-Youth-Employment?redirectedFrom=fulltext
More information on the seminar series is available on the CFG website.
Please contact Yiren Liu with any questions.
This event is sponsored by Conceptual Foundation Group (CFG) at the iSchool