Tanusree Sharma's Dissertation Defense
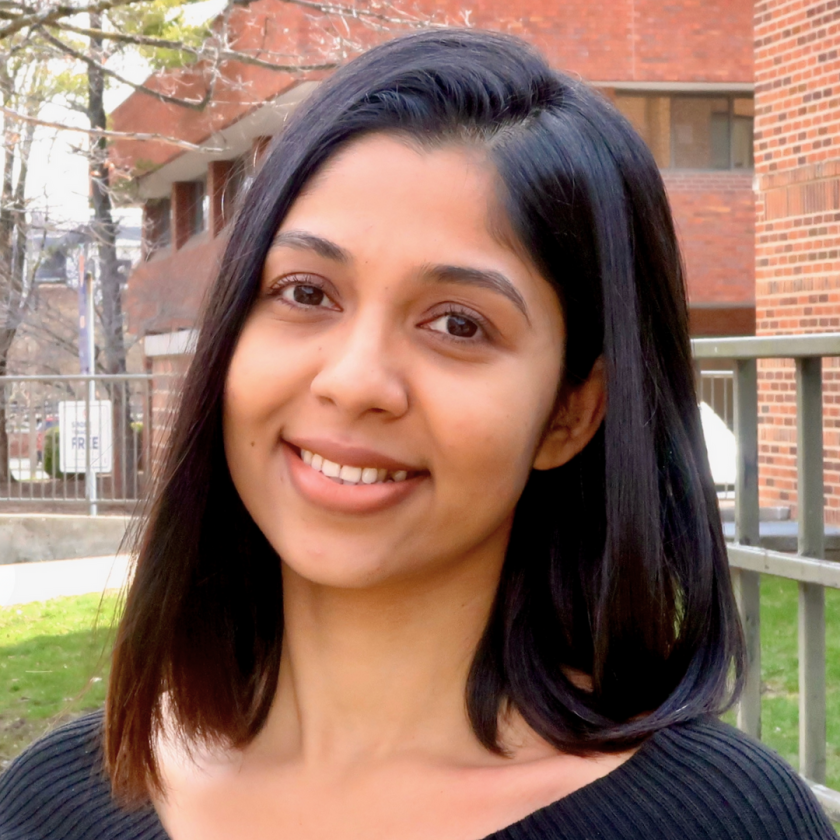
Tanusree Sharma will defend her dissertation, "Engaging People in Ethical AI Development: Design, Dataset Creation, and Decision-Making."
Online meeting number: 865 1003 4690
Password: 077228
Abstract
Major limitations of past AI development include but not limited to, the absence of thorough documentation and traceability in design, training with a small number of specific datasets, and lack of transparent and democratic decision-making processes for deploying AI models. These limitations could lead to adverse outcomes, such as discrimination, lack of privacy and inclusivity, and breaches of legal regulations. Underserved populations such as people with disabilities and minority groups, in particular, are disproportionately affected by these design decisions. In this dissertation, I employ qualitative, quantitative, and design methods to uncover end users' expectations for privacy and security in AI systems. I then develop methods to engage end users in the dataset creation and decision-making phases of AI development.
By examining user reactions to data minimization in AI systems, I find that users' assessments of privacy and security risks can be limited by bounded rationality. My analysis of the users-centric data minimization in the AI system design phase reveals how users reason about the necessity of data based on service quality, specific types of data, or volume and recency of data influenced by their mental capacity and available information. I then investigate how the data creation phase for AI development contributes to privacy risk, misrepresentation, and bias. To address ethical concerns, I developed a method for ethically creating a dataset with an underserved population (blind users) and built the first disability-first dataset, BivPriv, to support novel algorithm development for managing visual privacy and security. The findings highlight users' expectations to actively engage in the AI development lifecycle to be informed about how researchers or companies use the public data they share and how their contribution benefits AI systems.
Leveraging these insights, I designed Inclusive.AI, a democratic tool, equipped with decentralized governance mechanisms to engage end users in AI development stages enabling open access, and participation in shaping AI rules. This research highlights the importance of critically reflecting on when and how to engage end users in the AI development lifecycle. While engaging users is crucial, it is important to build a method for fair deliberation that considers the resilience of the decision-making process, the power structure among stakeholders, and the aggregation method used for gathering user preferences within practical constraints.