Jenna Kim's Dissertation Defense
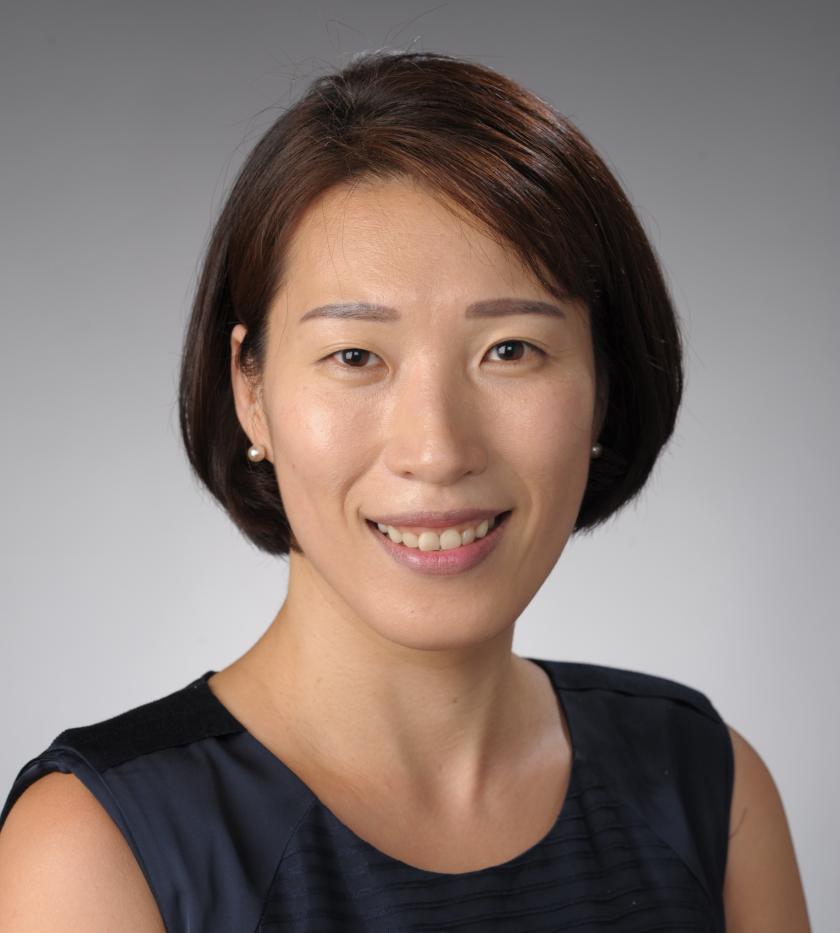
Jenna Kim will defend her dissertation, "Implementing Pre-Trained Language Modeling Approaches for Author Name Disambiguation."
Her committe includes Affiliate Associate Professor Jana Diesner, (chair and director of research) iSchool and professor at Technical University of Munich; Professor Bertram Ludäscher; Associate Professor Vetle Ingvald Torvik; and Assistant Professor Haohan Wang.
Questions? Contact Jenna Kim.
Abstract
Distinguishing between different authors who share the same names or identifying instances where different names refer to the same individual remains a persistent challenge in bibliometric research. This complexity impedes accurate cataloging and indexing in digital libraries, affecting the integrity of academic databases and the reliability of scholarship evaluation based on bibliographic data. Although various machine-learning (ML) methods have been explored to tackle the issue of author-name disambiguation (AND), traditional ML methods often fail to capture the subtle linguistic and contextual nuances essential for effective disambiguation. Moreover, while several studies have suggested that neural network models may surpass conventional ML models in AND tasks, the full potential of deep learning (DL) using advanced pre-trained language models (PLMs) like BERT has not been exhaustively examined.
This dissertation delves into applying PLMs for AND within scholarly databases and identifying its potential and limitations compared to traditional ML approaches. Specifically, this research aims to implement and evaluate three PLMs - BERT, MiniLM, and MPNet - against traditional ML algorithms and a neural network model across four established datasets and a newly introduced challenging dataset. The models are rigorously assessed using metrics including accuracy, precision, recall, and F1 score, emphasizing integrating abstract features from academic texts to enhance model comprehension and performance.
This dissertation contributes novel insights in several key areas. First, it pioneers the application of state-of-the-art PLMs in AND tasks, providing a comparative analysis with conventional ML and neural network approaches. Second, it broadens the features employed in existing studies by including abstract texts alongside the typical metadata records used in current AND research. Third, it integrates the workflows of various high-performing ML and DL methods for classification and clustering into an open-source framework.
The dissertation discusses the procedural benefits of using PLMs, such as the reduced need for manual feature extraction and selection, while addressing implementation challenges, including substantial computational demands and greater transparency in decision-making processes in implementing AND methods.
This dissertation advocates for adopting PLMs to resolve the complexities of AND, marking a significant advancement over traditional methods and paving the way for more sophisticated, context-aware computational solutions in the academic field. By elucidating the similarities and differences between various ML- and DL-based AND approaches, this research enhances the robustness of research findings that resolve author name ambiguity, thus supporting more accurate scientific analysis and decision-making based on bibliographic data.