Lanyu Shang's Dissertation Defense
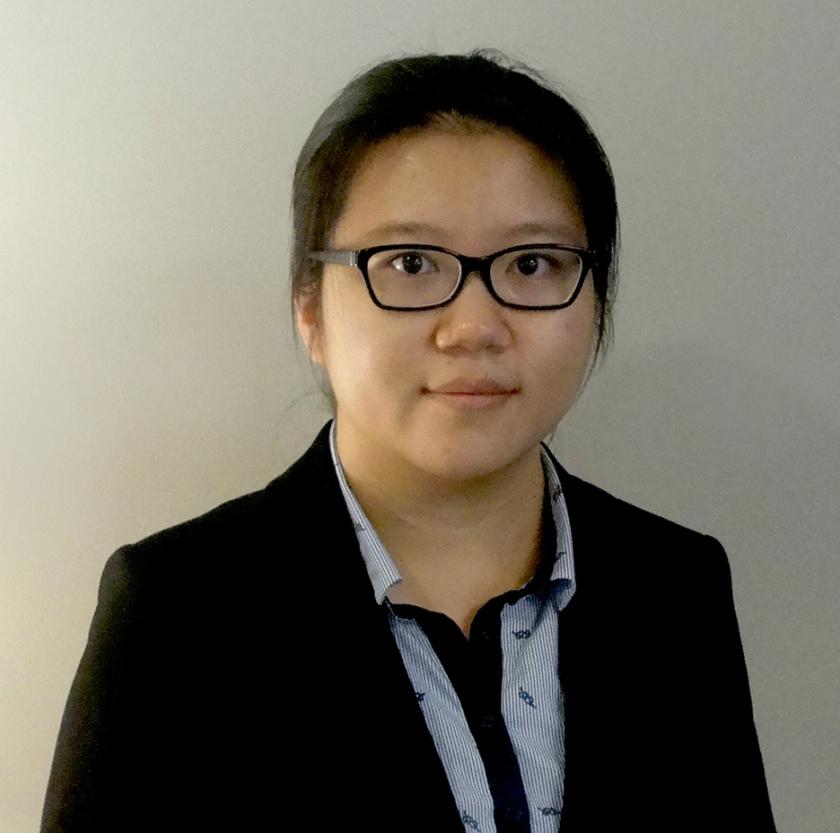
Lanyu Shang will defend her dissertation, "A Human-Centric Artificial Intelligence Approach Towards Equality, Well-Being, and Responsibility in Sustainable Communities."
Her doctoral committee includes Associate Professor Dong Wang, chair and director of research; Assistant Professor Jessie Chin; Professor Ximing Cai, Civil & Environmental Engineering; and Associate Professor, Na Wei, Civil & Environmental Engineering.
Abstract
This dissertation introduces a human-centric artificial intelligence for sustainable communities (HAI4SC) approach that harnesses the complementary strengths of artificial intelligence (AI) and human intelligence (HI) to foster equality, well-being, and responsibility. In particular, AI has shown superiority in processing large amounts of data, identifying latent patterns, and making predictions, which can help address the scalability and complexity of sustainability challenges. On the other hand, HI excels in providing context, domain expertise, and human-centric insights, which are essential for understanding the complex social and physical factors that influence community sustainability.
By harnessing the complementary strengths of HI and AI, this dissertation develops a holistic HAI4SC approach by addressing three fundamental challenges: multimodality, adaptability, and trustworthiness. First, we develop a multimodal information fusion system that introduces novel generative learning and cross-modal attention designs to seamlessly integrate multimodal content in enhancing information credibility in sustainable communities. Moreover, we design an adaptive cross-domain analytic framework that explicitly incorporates domain knowledge from well-studied source domains and learn domain-invariant representations that can be adapted to the target domain for improving the resilience and well-being of sustainable communities. Additionally, we develop a trustworthy social-physical knowledge distillation scheme that designs novel uncertainty-aware graph learning and attentive time series forecasting mechanisms to effectively model the diverse and uncertain human inputs from community stakeholders to strengthen community responsibility and sustainability.