Studying Science Scientifically Speaker Series: Liubov Tupikina
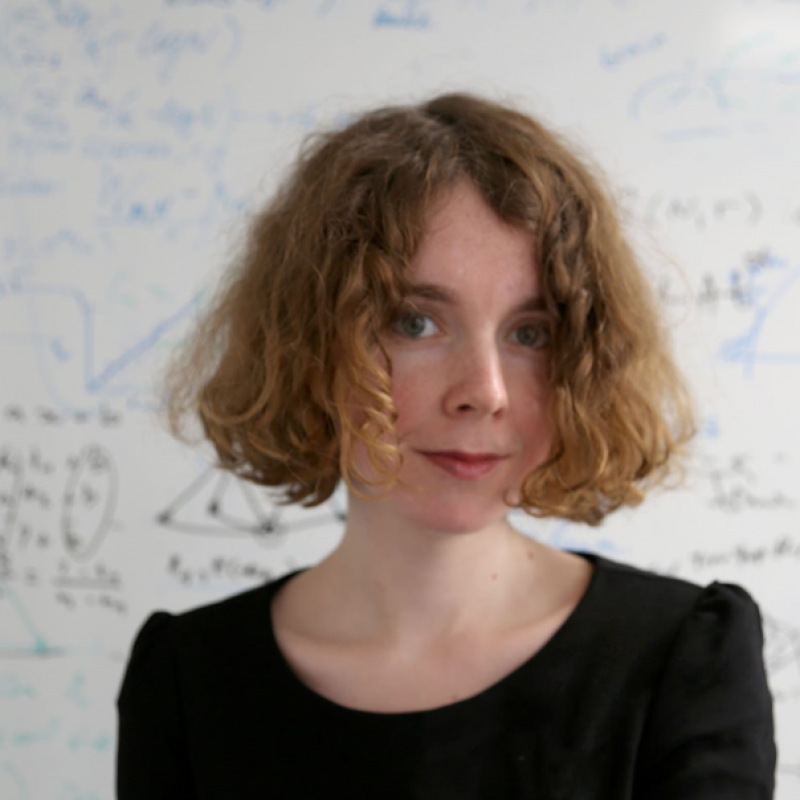
Liubov Tupikina will present, "Dissecting knowledge space: learning higher-order structures from data."
Liubov Tupikina (ITMO, Bell Labs, Paris Descartes LPI) is a researcher in computer science, mathematics and physics of complex systems. She has a PhD in theoretical physics, working on representation of dynamical systems using graph theory, and has worked on stochastic processes on graphs and hypergraphs. She now works on embeddings theory, low-dimensional data representations, higher-order mathematical structures representations of data encoded systems and hypergraphs encoding using algebraic theory (broadly explainable AI area). More information is on her website.
Abstract:
Active area of research in AI is the theory of manifold learning and finding lower- dimensional manifold representation on how we can learn geometry from data for providing better quality curated datasets. There are however various issues with these methods related to finding low-dimensional data representation of the data, the so-called curse of dimensionality. Geometric deep learning methods for data learning often include a set of assumptions on the geometry of the feature space. Some of these assumptions include pre-selected metrics on the feature space, usage of the underlying graph structure, which encodes the data points proximity. However, the later assumption of using a graph as the underlying discrete structure, encodes only the binary pair- wise relations between data points, restricting ourselves from capturing more complex higher-order relationships, which are often present in various systems. These assumptions on the data together with data being discrete and finite may cause some generalisation, which may create wrong interpretations of the data and models, which produce the embeddings of data itself (such as BERT and others).
The objective of our this talk will be to talk about several aspects of extraction of higher-order information from data, scientific data in particular. We will first talk about how to characterize the accuracy measure of the embedding methods using the higher-order structures. For this we explore the underlying graph assumption substituting it with the hypergraph structures and constructing knowledge hypergraph. Second, we aim to demonstrate the embedding characterization on the usecase of the example of some data with higher-order relations (such as arXiv open data).
About the speaker series:
The CIRSS Friday Speaker Series continues in Fall with a new theme of "Studying Science Scientifically: State of the Art and Prospects for the Science of Science.” With increasingly rich data sources, exciting new technologies for understanding natural language, and modeling methodologies adapted from diverse domains of scholarship, the opportunities to observe, measure, and model the structure and dynamics of the scientific enterprise abound as never before. We are inviting some of the leading thinkers and most innovative researchers to present at this talk series to illustrate the breadth of advances that have been made, and the many more yet to be made.
We meet most Fridays, 11am-noon Central time, on Zoom. Everyone is welcome to attend. More information, including upcoming speaker schedule and links to recordings, is available on the series website. For weekly updates on upcoming talks, subscribe to our CIRSS Seminars mailing list. Our Fall series is led by Timothy McPhillips and Yuanxi Fu, and supported by the Center for Informatics Research in Science and Scholarship (CIRSS) and the School of Information Sciences at the University of Illinois at Urbana-Champaign.
This event is sponsored by Center for Informatics Research in Science and Scholarship