Clara Belitz's Preliminary Exam
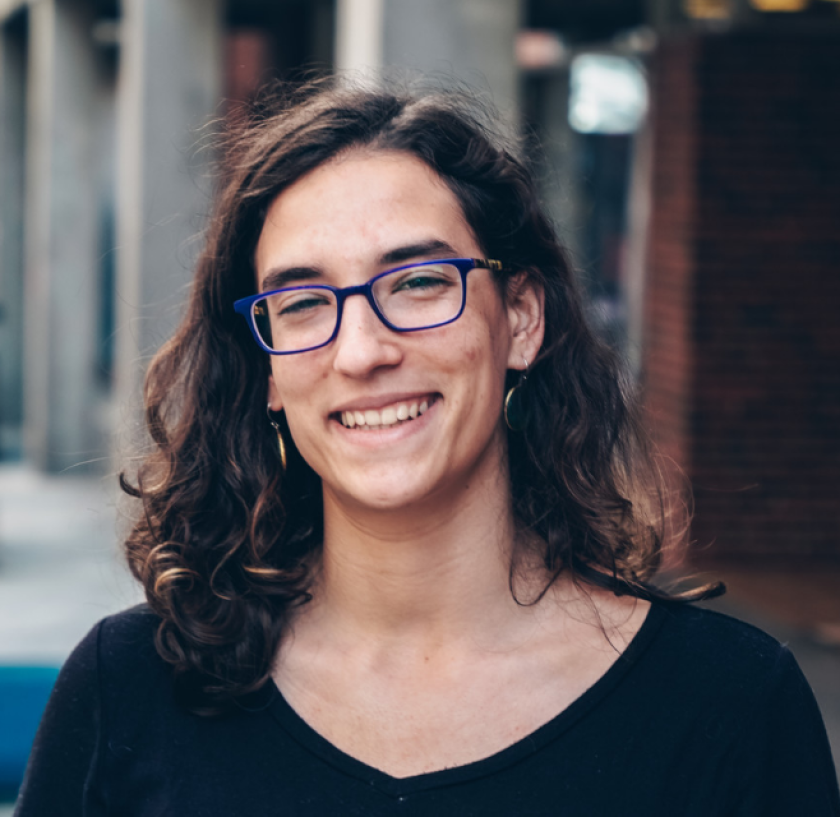
PhD student Clara Belitz will present her proposal defense, "Fair for whom? Investigating school identity, algorithmic fairness, and educational technologies." Her committee includes Assistant Professor Nigel Bosch (chair), Associate Professor Toby Beauchamp, Assistant Professor Jessie Chin, and Professor Jana Diesner (Technical University of Munich and Affiliate Associate Professor, iSchool at Illinois).
Abstract
Artificial intelligence (AI), as a subset of information and communication technologies, is rapidly proliferating across every sector of society, including in the classroom. Data-driven systems are frequently found in educational spaces in the form of adaptive tutoring systems and online learning environments. Previous research has shown that well-integrated technologies can augment good human teaching practices. How such integration is measured, however, is an evolving debate within both learning sciences and information sciences. Questions of ethics, equity, efficacy and accountability are topics of vigorous scientific conversation. Debate around scientific ethics is not a novel issue; stated scientific ideals are often in conflict with one another and require a balance in optimization goals—efficiency cannot come at the expense of ethical conduct, for example. Balancing complementary goals of “accuracy,” as measured by the (mis)match between observational labels and predicted outcomes, and “fairness,” as measured by one or more statistical metrics, requires thoughtful and reflexive choices about how scientists choose to measure these outcomes.
Previous work has focused more heavily on measuring accuracy, though the field of measuring fairness is growing rapidly. As such, I am interested in the question of measuring fairness and equity, inherently malleable human constructs. For example, what does it mean to center students in definitions of algorithmic justice? Using de-identified student surveys, school-provided demographic information, and trace data from student actions, I will explore dimensions of fairness and demographic alignment in algorithmic justice questions. In addition, I will pursue interviews with students to ask them, as the least powerful but arguably most impacted individuals in the algorithmic tutoring ecosystem, about their own relationship to these technologies and the way their data is used. Do the AI-driven technologies tend to deliver on their promises for education? If yes, are these improvements experienced equitably across different groups in the school system? According to whom? If no, what improvements could be made?