Tianxin Wei
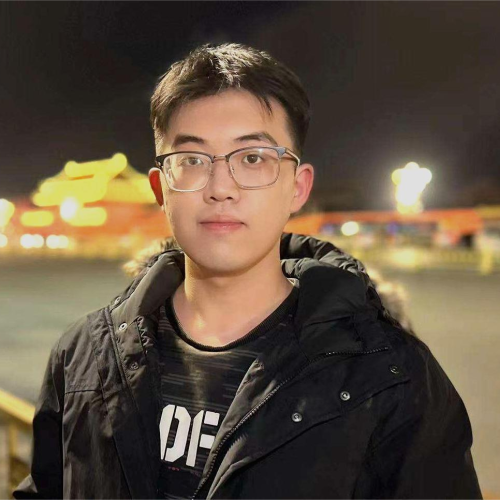
Doctoral Student
PhD, Information Sciences, Illinois (in progress)
BE, Computer Science, University of Science and Technology of China
Research focus
My research focuses on bridging the gap between transferability and personalization in machine learning, particularly in large language models (LLMs), with applications across modalities and disciplines.
Honors and Awards
- 2025 KDD Outstanding Reviewer
- 2024 University Nomination (Top3) for Apple Scholar in AI/ML
- 2024 Amazon Post-internship Fellowship
- 2023 Conference Presentation Award UIUC
- 2023 ICML Grant Award
- 2023, 2022 NeurIPS Scholar Award
- 2021 SIGIR Best Paper Honorable Mention
Advisor
Publications & Papers
T. Wei, Y. Chen, X. He, W. Bao, J. He. Connecting Domains and Contrasting Samples: A Ladder for Domain Generalization. KDD 2025
M. Ai*, T. Wei*, Y. Chen, Z. Zeng, R. Zhao, G. Varatkar, B. Darvish Rouhani, X. Tang, H. Tong, J. He. ResMoE: Space-efficient Compression of Mixture of Experts LLMs via Residual Restoration. KDD 2025
B. Jin, H. Zeng, G. Wang, X. Chen, T. Wei, R. Li, Z. Wang, Z. Li, Y. Li, H. Lu, S. Wang, J. Han, X. Tang. Language Models as Semantic Indexers. ICML 2024
T. Wei, B. Jin, R. Li, H. Zeng, Z. Wang, J. Sun, Q. Yin, H. Lu, S. Wang, J. He, X. Tang. Towards Unified Multi-Modal Personalization: Large Vision-Language Models for Generative Recommendation and Beyond. ICLR 2024
H. Zeng, C. Luo, B. Jin, S. M. Sarwar, T. Wei, H. Zamani. Scalable and Effective Generative Information Retrieval. WWW 2024
W. Bao*, T. Wei*, H. Wang, J. He. Adaptive Test-Time Personalization for Federated Learning. NeurIPS 2023
T. Wei, Z. Guo, Y. Chen, J. He. MLP Fusion: Towards Efficient Fine-tuning of Dense and Mixture-of-Experts Language Models. ICML 2023
T. Wei, Y. You, T. Chen, Y. Shen, J. He, Z. Wang. Augmentations in Hypergraph Contrastive Learning: Fabricated and Generative. NeurIPS 2022
T. Wei, J. He. Comprehensive Fair Meta-learned Recommender System. KDD 2022
T. Wei, F. Feng, J. Chen, Z. Wu, J. Yi, X. He. Model-Agnostic Counterfactual Reasoning for Eliminating Popularity Bias in Recommender Systems. KDD 2021