Qingxiao Zheng's Dissertation Defense
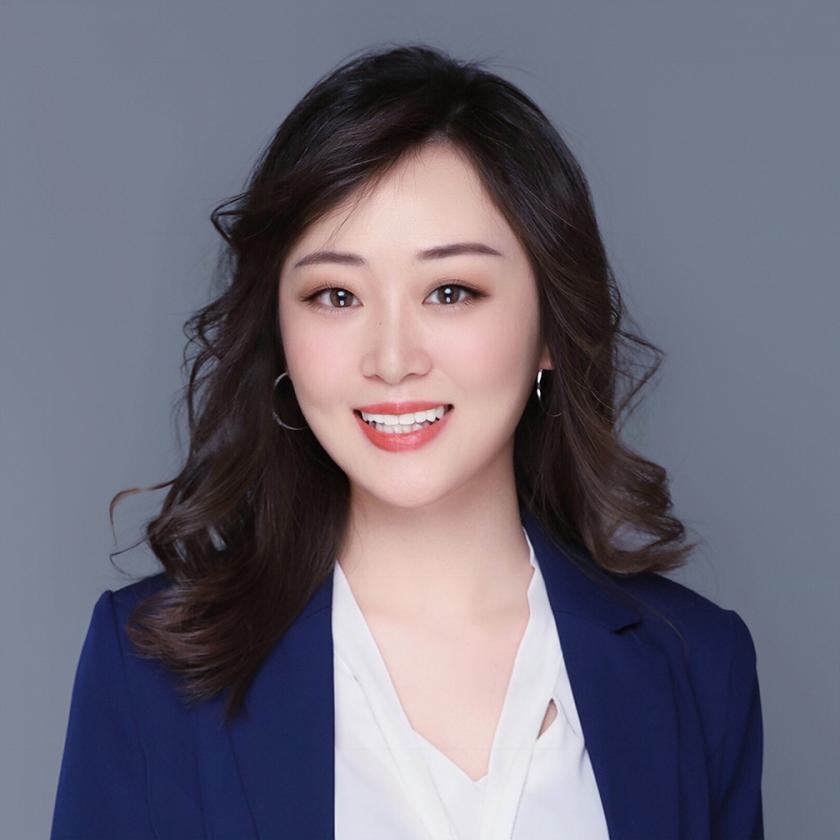
Qingxiao Zheng will defend her dissertation, "Shifting Paradigms in the UX Evaluation of Human-AI Interaction: From Dyadic to Monadic Designs".
Her committee includes Associate Professor Yun Huang, chair, School of Information Science; Professor Mike Yao, Institute of Communications Research; Associate Professor Yang Wang, School of Information Sciences; and Assistant Professor Nigel Bosch, School of Information Sciences.
Abstract
With generative AI, we are entering a new era where individuals without coding backgrounds can become creators of AI-based solutions for delivering different services. For example, business owners can define AI chatbots to answer customers’ inquiries, and legal professionals can tailor AI to draft, review, and manage legal documentation. However, there is a lack of a systematic framework to evaluate the UX of human-AI collaboration in these interactions.
This thesis identifies a paradigm shift in the UX evaluation of human-AI interaction. Prior to generative AI, UX research began with examining dyadic interactions between end users and AI, progressively expanding to polyadic interactions, where AI mediates between end users adopting multi-stakeholder perspectives (e.g., service providers and service consumers). The evaluation of these interaction modalities often considers user satisfaction, linguistic features, and task completion rate, and the challenges that mainly arise as a result of the uncertainty and complexity of AI. With the advent of generative AI, individuals with minimal AI literacy can become creators, introducing a new modality–monadic interaction, which emphasizes the unity and feedback loop between AI systems and their creator-users. These creator-users actively participate in defining and refining the AI's functions, allowing both to adapt and evolve. Although humans and AI systems can leverage their respective strengths to achieve better outcomes than either could independently, assessing how well AI aligns with creators’ values and intentions poses significant challenges, particularly when creators' norms deviate from broader societal standards.
This dissertation further introduces a UX evaluation framework, called EvalignUX (evaluating alignment of UX), designed to guide the evaluation of the three interaction modalities, while maintaining the alignment focus. First, I synthesized the literature on UX research in conversational human-AI interaction before the popularity of generative AI and proposed four dimensions for evaluating dyadic and polyadic interactions: linguistics and prosodics quality, task performance, socio-emotional intelligence, and ethics and risks. Each dimension includes several evaluation metrics, such as word variation, sense of control, perceived warmth and competence, and security perception. These evaluation metrics can serve as a design material to enhance UX effectively.
I then extended the EvalignUX framework to include monadic interactions through two case studies. The first case demonstrates creator-AI interaction within a multi-stakeholder service, where public service providers customize their AI to address community members’ queries. The second case illustrates creator-AI interaction for self-defined services, where creators possess diverse domain expertise and require AI to provide customized documentation for individual use.
The proposed EvalignUX framework can assist UX researchers and design tool makers in addressing the challenges of evaluating AI systems in a responsive fashion, from dyadic to monadic interactions. The thesis concludes with future research toward more aligned AI with boundary awareness.