Weakly Supervised Graph Neural Networks
Time Frame
Total Funding to Date
Investigator
- Jingrui He
Graph Neural Networks have proven to be a powerful tool for harnessing graph data, which is widely used for representing rich relational information in multiple areas. However, the performance of graph neural networks largely depends on the amount of labeled data, which is subject to an expensive and time-consuming annotation process. This creates data without labels, or a label scarcity.
This project focuses on building high-performing graph neural networks in the presence of label scarcity. This project generates a suite of new models, algorithms and theories for constructing high-performing graph neural networks with weak supervision, and for understanding the benefits of weak supervision from a theoretical perspective. It advances state-of-the-practice for graph neural networks by significantly reducing the need for large amount of labeled data.
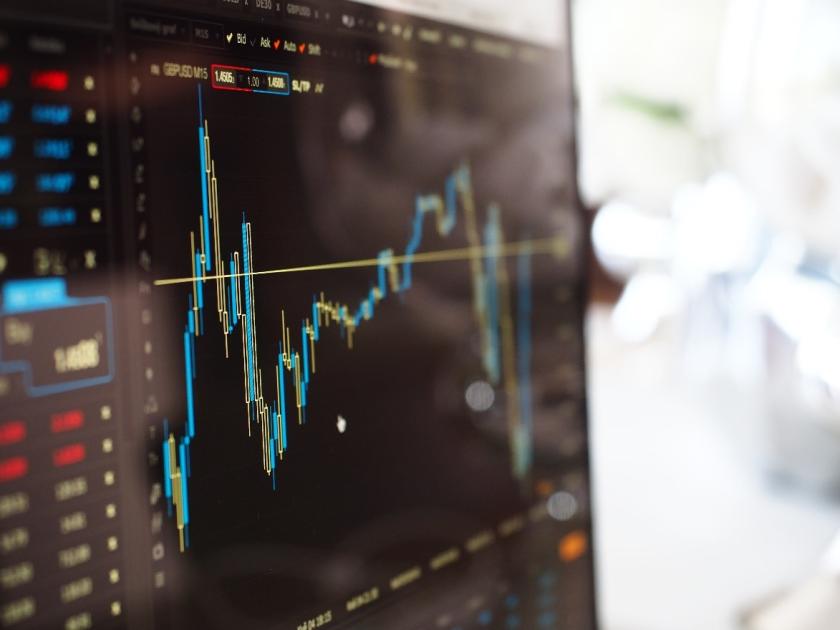
Personnel
Funding Agencies
- National Science Foundation, 2021 – $149,921.00