Haohan Wang
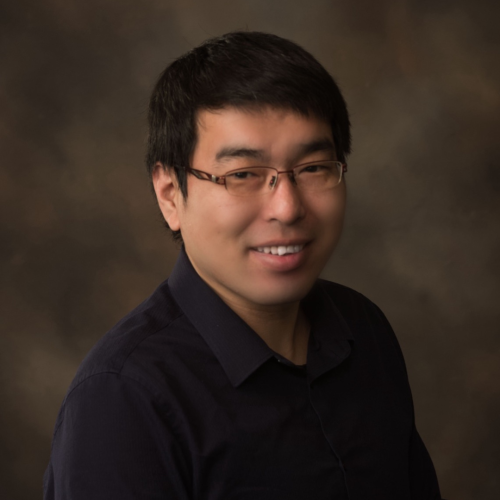
Assistant Professor
PhD, Computer Science, Carnegie Mellon University
Other professional appointments
- Organizer, Trustworthy Machine Learning Initiative
Research focus
Development and understanding of trustworthy machine learning methods with a focus on using the methods to understand biological and medical problems better, where the reliability of the methods is the key.
Honors and Awards
- Top 50 AI+X Rising Young Scholars by Baidu. Inc., 2022
- Youth Outstanding Paper Award by WAIC, 2021
- Next Generation: Rising Stars in Biomedicine by the Broad Institute of MIT and Harvard, 2019
- Most Influential Data Science Research Papers by media in ODSC, 2018
Biography
Haohan Wang is an assistant professor in the School of Information Sciences at the University of Illinois Urbana-Champaign. His research focuses on the development of trustworthy machine learning methods for computational biology and healthcare applications, such as decoding the genomic language of Alzheimer's disease. In his work, he uses statistical analysis and deep learning methods, with an emphasis on data analysis using methods least influenced by spurious signals (features that are statistically associated with the target but not causal). In 2019, Wang was recognized as the Next Generation in Biomedicine by the Broad Institute of MIT and Harvard because of his contributions in dealing with confounding factors with deep learning. Wang earned his PhD in computer science through the Language Technologies Institute of Carnegie Mellon University.
Courses currently teaching
Office hours
By appointment, please contact professor
Publications & Papers
Wang, Haohan, Zeyi Huang, Xindi Wu, and Eric P. Xing. "Toward Learning Robust and Invariant Representations with Alignment Regularization and Data Augmentation." KDD 2022. (https://arxiv.org/abs/2206.01909)
Wang, Haohan, Zeyi Huang, Hanlin Zhang, Yong Jae Lee, and Eric Xing. "Toward Learning Human-aligned Cross-domain Robust Models by Countering Misaligned Features." In The 38th Conference on Uncertainty in Artificial Intelligence. 2022. (https://arxiv.org/abs/2111.03740)
Wang, Haohan, Zeyi Huang, Dong Huang, Yong Jae Lee, and Eric P. Xing. "The Two Dimensions of Worst-Case Training and Their Integrated Effect for Out-of-Domain Generalization." In Proceedings of the IEEE/CVF Conference on Computer Vision and Pattern Recognition, pp. 9631-9641. 2022. (https://arxiv.org/abs/2204.04384)
Wang, Haohan, Zeyi Huang, Eric P. Xing, and Dong Huang. "Self-challenging improves cross-domain generalization." In European Conference on Computer Vision, pp. 124-140. Springer, Cham, 2020. (https://arxiv.org/abs/2007.02454)
Wang, Haohan, Xindi Wu, Zeyi Huang, and Eric P. Xing. "High-frequency component helps explain the generalization of convolutional neural networks." In Proceedings of the IEEE/CVF Conference on Computer Vision and Pattern Recognition, pp. 8684-8694. 2020. (https://arxiv.org/abs/1905.13545)
Wang, Haohan, Oscar L. Lopez, Wei Wu, and Eric P. Xing. "Gene Set Priorization Guided by Regulatory Networks with p-values through Kernel Mixed Model." In International Conference on Research in Computational Molecular Biology, pp. 107-125. Springer, Cham, 2022. (https://link.springer.com/chapter/10.1007/978-3-031-04749-7_7)
Wang, Haohan, Bryon Aragam, and Eric P. Xing. "Trade-offs of Linear Mixed Models in Genome-Wide Association Studies." Journal of Computational Biology 29, no. 3 (2022): 233-242. (https://arxiv.org/abs/2111.03739)
Wang, Haohan, Benjamin J. Lengerich, Bryon Aragam, and Eric P. Xing. "Precision Lasso: accounting for correlations and linear dependencies in high-dimensional genomic data." Bioinformatics 35, no. 7 (2019): 1181-1187. (https://academic.oup.com/bioinformatics/article/35/7/1181/5089232?ref=https://giter.site)
Wang, Haohan, Zhenglin Wu, and Eric P. Xing. "Removing confounding factors associated weights in deep neural networks improves the prediction accuracy for healthcare applications." In BIOCOMPUTING 2019: Proceedings of the Pacific Symposium, pp. 54-65. 2018. (https://www.worldscientific.com/doi/abs/10.1142/9789813279827_0006)
Wang, Haohan, and Bhiksha Raj. "On the origin of deep learning." 2017 (https://arxiv.org/abs/1702.07800)
Presentations
"Toward Trustworthy AI-diagnosis of Alzheimer's Disease from MRI" invited talk at Stanford CNS Lab in July 2022
"Toward a Principled Understanding of Robust Machine Learning Methods
and Its Connection to Multiple Aspects" invited talk at TrustML Young Scientist Seminar at RIKEN Center for Advanced Intelligence Project (AIP) (https://www.youtube.com/watch?v=sAQvgIiyLAU) in July 2022
"Robust Computer Vision Techniques Help Identify Subtypes of Alzheimer’s Disease" invited talk at Department of Biomedical Informatics Colloquium Series, University of Pittsburgh in Nov. 2021
"Robust Machine Learning with Emphasis on Countering Spurious Features" at Data Science Initiative, Brown University in March 2021
"High Frequency Component Helps Explain the Generalization of CNN" at Aggregate Intellect in Jan. 2021 (https://www.youtube.com/watch?v=Njpip9-_Xug)
"Towards Trustworthy Machine Learning Inspired by High-frequency Data" at Robotics Institute, Carnegie Mellon University in Nov. 2020
"Towards Trustworthy Machine Learning for Scientific Discovery" at Doctoral Symposium at ACM Conference on Health, Inference, and Learning in July 2020
"Learning Deconfounded Representations and Applications in Genetic Data" at Center of Excellence for Computational Drug Abuse Research in Feb. 2020
"Dealing with Confounding Factors in Deep Neural Networks" at Next Generation in Biomedicine Symposium, the Broad Institute in Sept. 2019