Yang Zhang
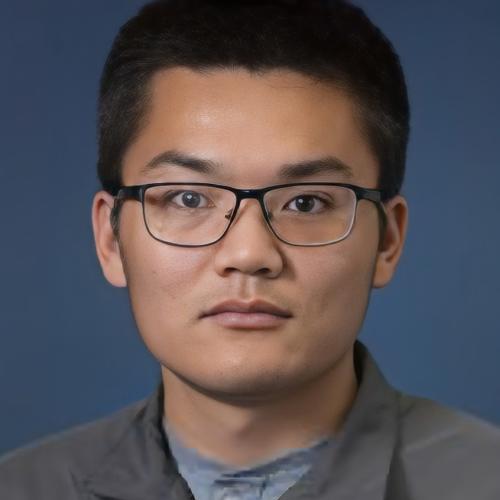
Teaching Assistant Professor
PhD, Computer Science and Engineering, University of Notre Dame
Other professional appointments
- Graduate Faculty, Graduate College
- Senior Researcher Affiliate, Social Sensing & Intelligence Lab
Research focus
Human-centered Artificial Intelligence (AI) and Human-AI Interaction and Collaboration. Utilizing crowd intelligence to effectively design, configure, and troubleshoot the AI models to improve the performance, fairness, explainability, and accountability of the AI models.
Honors and Awards
- Best Paper Award, ACM/IEEE ASONAM 2022
- Best Paper Honorable Mention, IEEE SMARTCOMP 2022
- Outstanding Graduate Research Assistant Award, University of Notre Dame
- Video Presentation Award, ACM/IEEE IWQoS 2020
- W. J. Cody Associates, Argonne National Laboratory
- IEEE Student Travel Award, IEEE BigData 2019
- IEEE Student Travel Award, IEEE BigData 2018
- Data Science Scholar Fellowship, Indiana University
- First Class Scholarship, Wuhan University
Biography
Yang is working as a teaching assistant professor in the School of Information Sciences (iSchool) at the University of Illinois Urbana-Champaign (UIUC). Yang is also affiliated with the Social Sensing & Intelligence Lab at UIUC as a senior researcher. Before that, Yang worked as a postdoctoral research associate for the iSchool at UIUC. Yang received his Ph.D. in Computer Science and Engineering from the University of Notre Dame. Yang also worked as the W. J. Cody research associate at Argonne National Laboratory.
Publications & Papers
Y. Zhang, R. Zong, L. Shang, H. Zeng, Z. Yue, and D. Wang. On Optimizing Model Generality in AI-based Disaster Damage Assessment: A Subjective Logic-driven Crowd-AI Hybrid Learning Approach. Proceedings of the International Joint Conference on Artificial Intelligence (IJCAI). Macao, SAR, 2023.
Y. Zhang, L. Shang, R. Zong, H. Zeng, Z. Yue, and D. Wang. CollabEquality: A Crowd-AI Collaborative Learning Framework to Address Class-wise Inequality in Web-based Disaster Response. Proceedings of the International World Wide Web Conference (TheWebConf). Austin, Texas, 2023.
Y. Zhang, Z. Kou, L. Shang, H. Zhen, Z. Yue, and D. Wang. A Crowd-AI Duo Relational Graph Learning Framework Towards Social Impact Aware Photo Classification. Proceedings of Thirty-Seven AAAI Conference on Artificial Intelligence (AAAI), 2023, Washington, DC, 2023. [Acceptance Rate: 19.6%]
Y. Zhang, R. Zong, L. Shang, Z. Kou, and D. Wang. An Active One-Shot Learning Approach to Recognizing Land Usage from Class-wise Sparse Satellite Imagery in Smart Urban Sensing. Elsevier Knowledge-Based Systems (KBS). [Impact Factor: 8.038]
Y. Zhang, R. Zong, L. Shang, and D. Wang. On Coupling Classification and Super-Resolution in Remote Urban Sensing: An Integrated Deep Learning Approach: A Deep Duo-Task Learning Approach. IEEE Transactions on Geoscience and Remote Sensing (TGRS). [Impact Factor: 5.6]
Y. Zhang, R. Zong, L. Shang, Z. Kou, and D. Wang. CrowdNAS: A Crowd-guided Neural Architecture Searching Approach to Disaster Damage Assessment. In Proceedings of the ACM Conference on Computer-Supported Cooperative Work and Social Computing (CSCW). Virtual Conference, 2022.
Y. Zhang, R. Zong, L. Shang, Z. Kou, H. Zeng, and D. Wang. CrowdOptim: A Crowd-driven Neural Network Hyperparameter Optimization Approach to AI-based Smart Urban Sensing. Proceedings of the ACM Conference on Computer-Supported Cooperative Work and Social Computing (CSCW). Virtual Conference, 2022.
Y. Zhang, L Shang, R. Zong, Z. Wang, Z. Kou, and D. Wang. StreamCollab: A Streaming Crowd-AI Collaborative System to Smart Urban Infrastructure Monitoring in Social Sensing. Proceedings of the AAAI Conference on Human Computation and Crowdsourcing (HCOMP), Virtual Conference, 2021. [Acceptance Rate: 26.0%]
Y. Zhang, R. Zong, Z. Kou, L. Shang, and D. Wang. On Streaming Disaster Damage Assessment in Social Sensing: A Crowd-driven Dynamic Neural Architecture Searching Approach. Elsevier Knowledge-Based Systems (KBS). [Impact Factor: 8.038]
Y. Zhang, R. Zong, Z. Kou, L. Shang, and D. Wang. CollabLearn: An Uncertainty-Aware Crowd-AI Collaboration System for Cultural Heritage Damage Assessment. IEEE Transactions on Computational Social Systems (TCSS). [Impact Factor: 5.357]